
Since people invented writing, communications technology has become steadily more high-bandwidth, pervasive and persuasive, taking a commensurate toll on human attention and cognition. In that bandwidth war between machines and humans, the machines’ latest weapon is a class of statistical algorithm dubbed “deep AI.” This computational engine already, at a stroke, conquered both humankind’s most cherished mind-game (Go) and our unconscious spending decisions (online).
AI, Our Ultimate Moral Censor
This month, finally, we can read how it happened, and clearly enough to do something. But I’m not just writing a book review, because the interaction of math with brains has been my career and my passion. Plus, I know the author. So, after praising the book, I append an intellectual digest, debunking the hype in favor of undisputed mathematical principles governing both machine and biological information-processing systems. That makes this article unique but long.
Bringing AI to the World
“Genius Makers: The Mavericks Who Brought AI to Google, Facebook, and the World” is the first book to chronicle the rise of savant-like artificial intelligence (AI), and the last we’ll ever need. Investigative journalist Cade Metz lays out the history and the math through the machines’ human inventors. The title, “Genius Makers,” refers both to the genius-like brilliance of the human makers of AI, as well as to the genius-like brilliance of the AI programs they create. Of all possible AIs, the particular flavor in the book is a class of data-digestion algorithms called deep learning. “Deep” as in “many layers of complexity,” not deep as in “profound and simple.” There’s a big difference.
Metz’s book is a ripping good read, paced like a page-turner prodding a reader to discover which of the many genius AI creators will outflank or outthink the others, and how. Together, in collaboration and competition, the computer scientists Metz portrays are inventing and deploying the fastest and most human-impacting revolution in technology to date, the apparently inexorable replacement of human sensation and choice by machine sensation and choice. This is the story of the people designing the bots that do so many things better than us. Metz shows them at their most human.
I won’t burden you with too many of Metz’s personal observations about these great minds but for a few illustrative examples. The father of deep learning, Geoff Hinton, dislikes “too many equations” (that’s my kind of scientist). Mark Zuckerberg, the founder of Facebook has a speech tic. Google’s founder, Larry Page, believes technology is good.
But this is also the story of the mathematical tools that those people discover or invent, math that will long outlast them. These technologists discover new principles before they program them into computers. Then, they tune up improvements until their creations sing or take over the world. I recognize them as my tribe and I thrill at their triumphs. I’ve been there too.
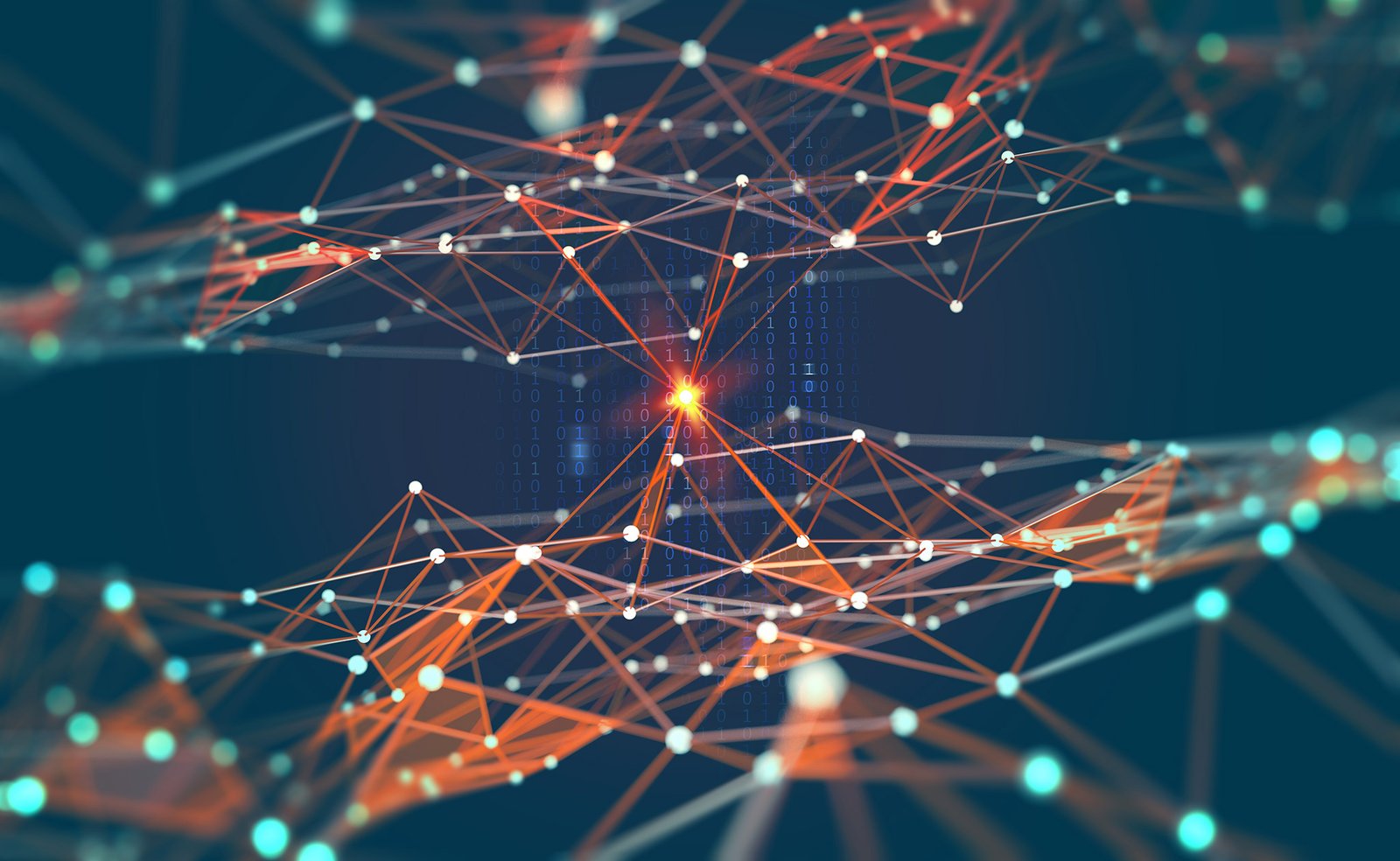
Along with the people and their math, Metz includes the kind of potent business insight he’s been long known for — the kind obvious only in hindsight. For example, he points out that Microsoft forced its own AI researchers to use clunky, closed Windows programming platforms. That rule so frustrated those researchers that they left Microsoft, and thus left Microsoft behind in the AI race.
Metz’s chapter titles convey his sense of drama. The chapters introducing the players have titles like Promise, Rejection, Breakthrough, Ambition, Rivalry and Hype. The chapters about AI goes rogue are similarly telling: Deceit, Hubris, Bigotry, Weaponization and Impotence.
Tech Triumphs
“Genius Makers” describes the explosion of AI as yet another California Gold Rush, saturated in hype and money much like the state’s earlier movie, aerospace, cult and startup booms. For generations, California has specialized in mating money with persuasion, technology and scale. It moved fast and broke things.
Metz records AI’s recent history with the players. Everyone agrees these events were driven, or rather incentivized, by the universal pressures of money and persuasion in all its forms: publicity, reputation, image, hype, power.
Certain behaviors emerge when money meets persuasion: hucksterism, overselling, a focus on pleasing funders. Because money tends to flow toward anticipated profits more than demonstrated usefulness, those who wish to bend it lean toward shoddy metrics and calculation tricks. In fact, that bending is a law of information physics. Much like glass, money has a refractive index on messages, bending them toward the source that paid their fare.
Both honest reporting (like Metz’s) and common sense tell us that most humans, companies and probably governments would act in the same narrow, self-serving ways as the people in this book. Using that insight, one can dispense with the particulars of who did what. Not to remove the human element, but to focus on the core question apart from all the hype and bogus claims: What is this technology, and how will it impact us?
The most important point is that AI is not based on brains at all. The best quote comes from computer scientist Alex Krizhevsky: “Deep learning should not be called AI … I went to grad school for curve-setting.” He points out that deep learning is really just a form of math called “non-linear regression.” Mathematical inference for complex statistics, not a brain at all.
But what a form of math deep learning is! It was founded on the most reliable scientific principles possible, those of thermodynamics and information theory (which share crucial concepts in common, such as entropy). Those twin principles doubly illuminate the target of an ultimate inference engine, so researchers could hit it directly.
The specific tasks set for AI varied from general to specific. The most general goal of AI, in common to all tasks, was to “learn” (i.e., map and distill) the underlying structure of a target data space upon exposure to its data. More specific tasks were to recognize examples from the target space, categorize them or use them to control future data.
Researchers took on practically every commercially viable or publicity-worthy task possible: face recognition, speech recognition, speech synthesis, speech translation, text translation, image classification, image analysis and image synthesis.
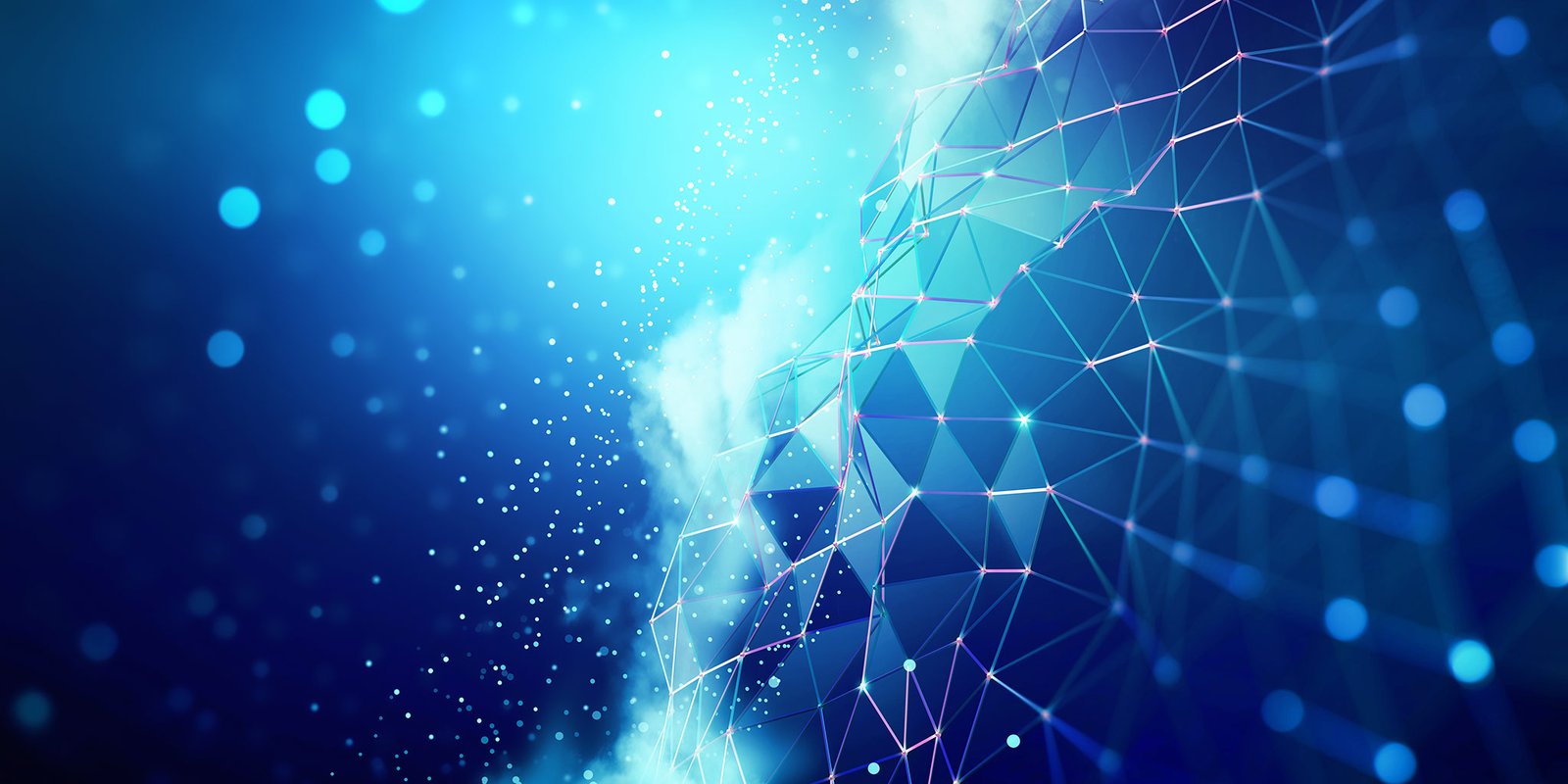
What makes a task commercially viable? Something humans aren’t good at. Humans are very good at seeing, hearing and touching the real world. The further from it or the more abstract the task, the worse we do. In general, AIs are the opposite. So, an AI that analyzes spreadsheets or computer programs might be profitable, but not one competing on our native turf — say, identifying crosswalks or talking sense because humans are cheap and plentiful and we do those well already. The most profitable AIs, and thus those likely to take over the world the fastest, replace humans at what they’re paid to do, like “picking” items out of Amazon crates (a problem now solved).
Although it’s typically not profitable to pay AIs to look at pictures (unless you’re Facebook), it can be profitable for AIs to show pictures to people. AIs are very good at observing what grabs people’s attention. Now, they can also synthesize fake, weirdly-interesting pictures and videos. Or they can choose which ads to show, which is where most of the money is made.
AI does two things well: recognition and control. In recognition, the input is data like an image, video or a sound, which its output describes later. Recognition is tricky for mathematical reasons because the so-called “curse of dimensionality” makes it hard to follow slopes and gradients, so they thus need lots of data to train.
In control, inputs and outputs are simultaneous, as the AI interacts with a continuous world: either a virtual game-world like Breakout or Quake, the real world of a grasper, drone or self-driving car. Stuck in 3-space, continuous control not only can use gradients, but it has to. Physical control is made difficult by physical effects like grit, momentum and lighting artifacts. Both recognition and control systems can suffer from the data disease of “overfitting,” a kind of invisible rut in which the AI learns to connect its data dots so well, too well, that it gets confused by new details.
Good training data was (and still is) essential to training any learning system, whether human or AI. The most efficient forms of learning, “supervised learning,” use hand-picked and hand-labeled data. Labels make learning easier because the AI only has to gather statistics for predicting the labels.
The more difficult task of “unsupervised learning” forces the AI to discover structure in the data on its own, without knowing what the humans think the answer is. Biological learning is unsupervised. In fact, my post-doctoral fellowship at the US National Institutes of Health resulted in a 1995 paper at the Neural Information Processing Systems (NIPS) conference explaining how the brain learns to correct itself using predictions.
The best training data is not evidence but math. In the rare cases when a task is completely rule-based, as with games like chess and Go, an AI can generate legal and legitimate examples internally much faster than it could gather data from outside. That means the AI can gain “experience” at hyperspeed, faster than any human could. Thus, the world Go championship now belongs to an AI, and will forever. The performance of the program AlphaGo became god-like after playing millions of games with itself, teaching itself from scratch using trial and error.
Next, after good training data is the algorithm that learns from it. The first AI, the 1958 Perceptron, just measured a few statistics. The next innovation added signals to reinforce a job well done. Multiple layers stacked up next, so that one layer fed the next. Those static recognition nets later learned to reconstruct sequences. Finally, all those methods were supercharged with statistical modeling and estimates of belief (“Bayesian priors”), which could fill in blank spots.
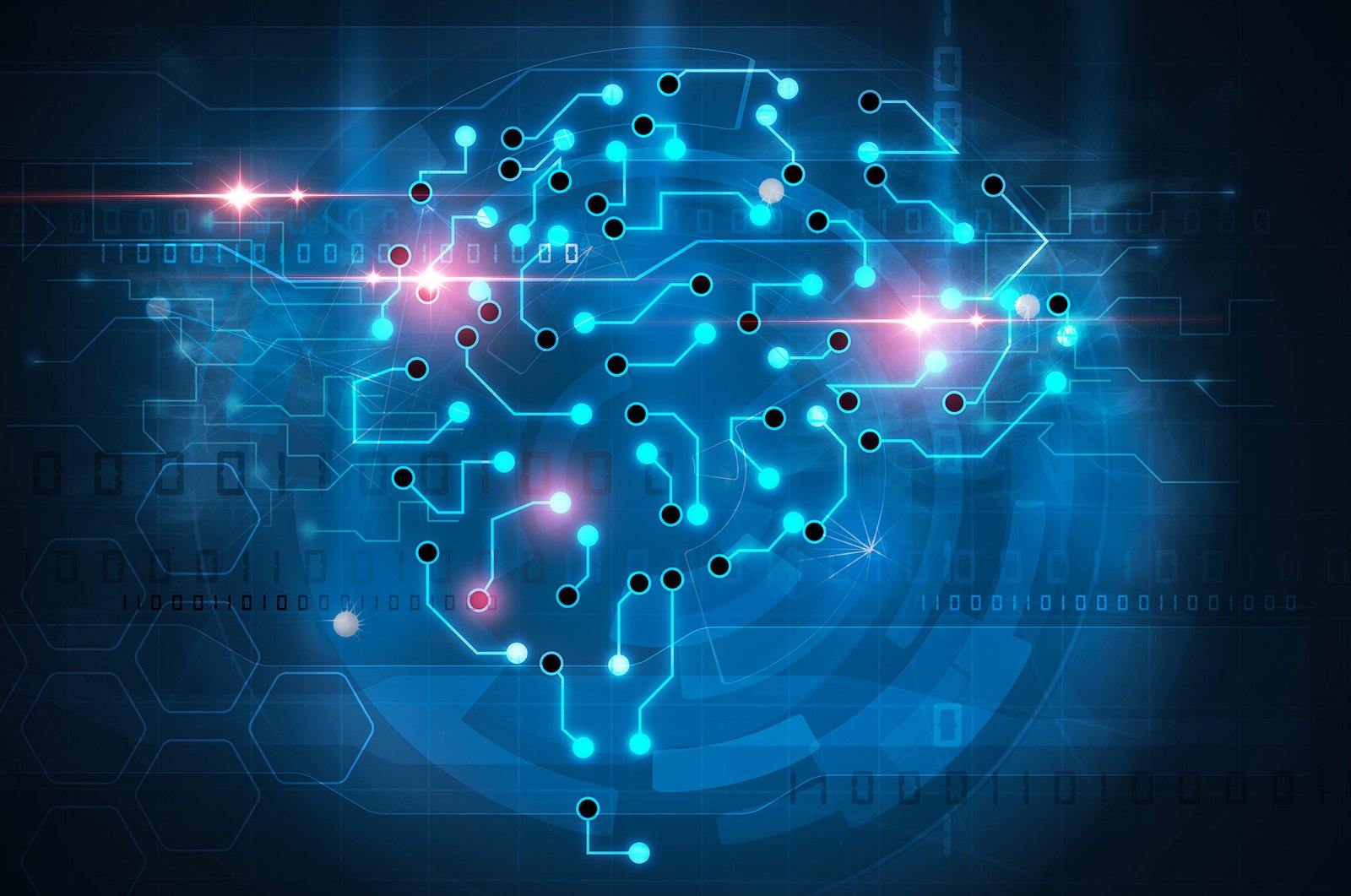
The laggard in AI is hardware. Even modern AIs are millions-fold less efficient than humans in using data and energy, which is why they need so much. So, companies save a lot of money by optimizing the hardware to match the task, like using special-purpose computer architectures, and even special-purpose chips.
Musk Sounds the Alarm, Marcus Calls Their Bluff
It is a testament to Metz’s deft writing that none of the dozens of geniuses he profiles looks bad in his book. But only two look really good, for standing up alone to speak the truth.
The loudest, most famous and probably most brilliant is Elon Musk. Alone among tech titans, Musk made his mint not in the virtual world of software, but in the physical world of recalcitrant materials and crushing forces: rocketry, electric cars and solar electricity. He takes on mother nature, not man-made protocols. He can’t bluff his way past artificial milestones like the software moguls can, so he has to know his physics cold. Anyone who can build a working rocket or a high-speed car has my attention when he talks about instability and explosion.
And talk he does. Musk knows how fast and furious runaway exponential growth can be, and he evidently warned a lot of bigshots, up close and personal, that scaling-up the automated manipulation of human beings is a very bad idea, capable of wiping out humanity: “One has to be thinking of ethical concerns the moment you start building the system.”
I only wish Musk hadn’t used the term “superintelligence” for the machines that might take over the world. That vague term plays into the myth that AI is intelligent like brains are. The immediate threat is not superintelligence but sub-intelligence, as a swarm of soulless, hidebound spreadsheets around the world overrule more and more human decisions about spending, hiring, lifesaving, imprisoning and causing war. Relative to human judgment, it’s possible that spreadsheets have already taken over the world. Spreadsheets are machines. And spreadsheets are the bosses of the robots and AIs, not the other way around.
The other bold critic of the AI boom is the neuroscientist (not merely neural-net expert) Professor Gary Marcus. He calls out the claim that AI works like brains because he knows that brains do something AIs can’t, which is to learn efficiently. He says, “Children can learn from tiny amounts of information.” Marcus makes a point I’ve tried to make for years: “Learning is only possible because our ancestors have evolved [innate] machinery for representing things like time, space, and enduring objects.” (I’ll explain how that works toward the end.)
The most scientifically pertinent observations in “Genius Makers” come not from AI’s promoters or apologists, but its detractors. Krizhevsky says AI can’t have mathematical intelligence, Marcus says it can’t have human intelligence and Musk says any intelligence will be dangerous. I say deep AI is approaching a mathematical optimum for three specific technical tasks: 1) navigating purely rule-driven hyperspaces like Go; 2) learning multilayered statistical structures like ontologies; and 3) learning low-dimensional dexterous robot control, like warehouse picking. It’s already better than humans could ever be. That’s the problem. That’s why I think those three geniuses are right.
The Bad News Now
Unfortunately, none of those three accomplishments are good for humans as a whole. The first takes away the most honored boardgame in history. The second find ways to distract and fool us more effectively. The third replaces low-paid human labor with even cheaper machine labor — everywhere soon.
Even after scrapping the distracting term “intelligence,” it’s clear something enormous has happened and will continue happening, as energy and hardware inefficiencies are optimized away.
AI’s thirst for data is something yet again because good training data must be flawless. But unsullied, gold-standard data corpuses are a thing of the past. Now, much of the content on platforms like Twitter is created by bots, not people. Most text on the web has been optimized to please Google’s so-called “quality score,” so it can’t be a reference for human communication, nor for anything else. There are only so many trustworthy data sources in the world and most of them are now corrupt. Even if we invent another, it still takes time to trust.
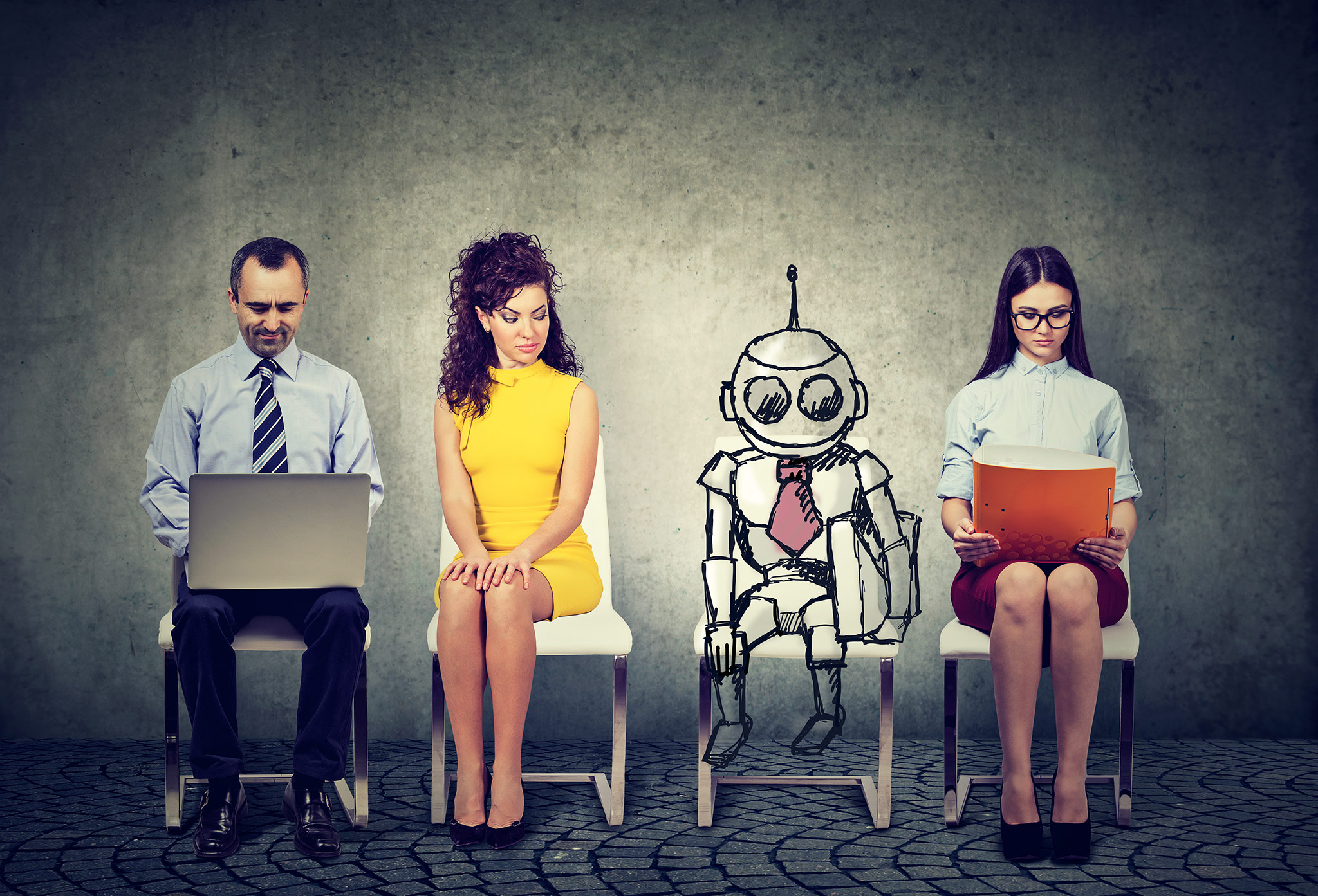
The most commercially successful hidden AIs have been auto-advertising and auto-interrupting algorithms. The most annoying are robo-calls, the bottom feeders. I estimate these calls cost the recipients tens of billions of dollars in wasted time, stress and attention, in order to yield the robo-deployers a tiny fraction of that value. AI makes robo-calls possible in three ways: AI picks your phone number from a list, it concocts the (fake) origin number to show you and it runs the interactive voice pretending to be human. Deep AI can only make those deceptions more effective.
Next up are phone menus and automated services that replace live human helpers with bots. Bots cost such a tiny fraction of what people cost, but they only deliver service half as good. That lopsided ratio seems like a net benefit to bean counters who don’t count human costs. To customers, it spells frustration or despair. Among the worst phone-mail offenders is the original phone company, Ma Bell. At the top end are retail voice bots like Alexa and Siri, which already sound too human for psychic safety (in which case their popularity is moot). Those, and the ads and the deepfakes, are the AIs that work.
The AIs that don’t work, and won’t ever work accurately enough for institutions to be honest about their performance, include face recognition, moderation algorithms to remove otherwise-profitable hate speech, medical diagnostic algorithms, educational-technology algorithms, hiring algorithms and therapy algorithms. Unfortunately, it’s economically and legally impossible for organizational sponsors to be honest about the inevitable failures of such programs.
One large organization profiled in “Genius Makers” claims to make information accessible and useful, but, in fact, it reflexively hides evidence of its own failures, selectively dissembles to manage its image and breaks serious promises to cozy up to power. That’s rational behavior, economically. That’s the problem.
Unavoidable Paradoxes
The “deep” part of deep learning isn’t even the technology’s multilayer statistical algorithms, but the intellectual contradictions it lays bare. Take these examples, for instance.
Can parasitic economies be permitted? Many jobs now involve “sales” — that is getting the attention of people and/or persuading them. When humans do it, that works fine because they can only distract, misrepresent and/or coerce so much. But as robo-calls and robo-scams get cheaper, more effective and harder to limit, the market price of interrupting and manipulating people goes to zero, so intrusions and deceptions multiply and peace of mind becomes progressively impossible. Now that machines can influence people so much and so well, there may be no way to stop them from overdoing it collectively. Micro-deceptions can be invisible when produced and consumed (thus hard to regulate), but they still add up in our brains. Economically, an economy of attention or deception is as unsustainable as an economy of organ harvesting. Death by a million milli-cuts.
What does it mean to disrupt communications? Communication systems work best when they change the most slowly because that lowers the uncertainty in meaning. Activities like rebranding, which redefine the words and images, overtly undermine the very contract of communication. Ever-shifting media and interfaces undermine it less obviously, but disruption still disrupts.
Is trust a bubble about to pop? Trust is quickly undermined and slow to rebuild because of its statistical sensitivity to errors and outliers. In particular, human trust in commercial activities — say, trust in printed money — has accumulated over centuries of human-to-human and human-to-shop interaction. Insofar as trust needs human interaction, its replacement by mere trustworthy markers will create a rickety fake system that bleeds out real trust, yet cannot restore it. The obvious villains are glitchy, stupid and venal AIs. But even a perfectly-working AI can’t convey human trust.
Is it fair to ignore edge cases? Being statistical, AIs are trained on the centroid and can’t accommodate the filigreed detail of diversity, nor can they know when they’ve encountered it. AI makes outliers truly invisible. That disempowers almost everyone, since everyone is an outlier somehow.
All physical representations are fake-able, but digital ones the most. In “Genius Makers,” computer scientist Ian Goodfellow says: “It’s been a little bit of a fluke, historically, that we’re able to rely on videos as evidence that something really happened.” The principle, “the more modifiable, the more fakeable” is true of every physical and virtual medium. If the trend of fakery continues, nothing on a screen will be trustworthy and much of paper will be suspect.
Is “human intelligence” about symbolic skills that set us apart from other animals or about neuromechanical infrastructure shared in common? What AI does well are things that make us proud of human brains: memory, symbolic analysis, language, categorization, gameplay and story. Animal brains do none of those things. But our brains’ informational needs for authenticity, autonomy, continuity and diversity are effectively animal. Symbolic activities, which clunkily use the sympathetic nervous system, tend to damage neural bandwidth and mental peace (see below). On the other hand, most animal activities like socializing, moving and resting are good for us.
How does one deal with conceptual paradoxes like these? We are facing not just conflicting evidence, but conflicting first principles. So, we have to start from scratch, find which principles are really first and treat them accordingly. For example, Albert Einstein voted for thermodynamics as the most unshakeable physical theory, even over his own theory of relativity.
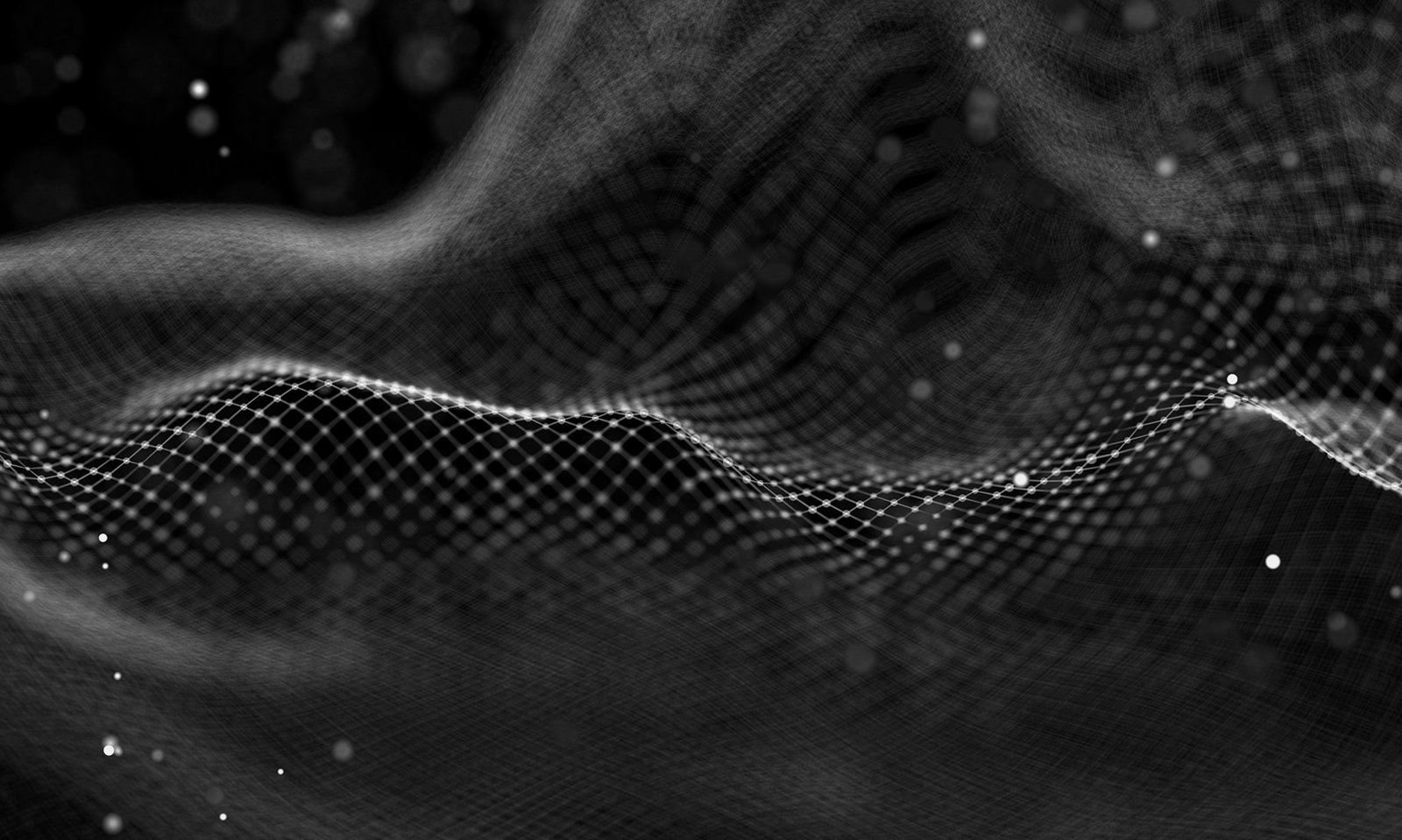
Ordering first principles is where physicists excel in general. So, below, I’ll spend two short sections on math, before redescribing life and brains from the ground up. This exercise is something Silicon Valley might call a clean-room reinstallation of our knowledge base. The language has to be technical, but you can skip those sections if you want.
However wonderful the arc of nervous system evolution proves to be, at the end of this exercise, we’ll discover that our best-credentialed, best-paid computer scientists collectively (including me) have made the grossest, dumbest goof that software types can ever make: We forgot about the hardware on which our software runs.
My Life Building Technology
Cade Metz might have been born to write this book. In which case, I was born to write this analysis. Here’s my case.
My parents were both nuclear physicists. I grew up as a radio and electronic hardware hacker in Silicon Valley before we called it either hardware, hacking or Silicon Valley. My kid brother had a patent in high school. In 1978, Ed and I pirated the public-address system at Menlo-Atherton High School to broadcast a bootleg announcement canceling final exams. I worked summers at the high-tech plastics factory Raychem, whose rubble now supports Facebook’s galaxy-sized headquarters.
Like most of the players in Metz’s book, I’m a middle-aged, white male. I try to be sensitive to the most common strains of human racism, and I’m deeply concerned about the algorithmic versions.
After college, I worked at the original Bell Labs as a “laser jock” doing nuclear physics with plasmas. There, I heard John Hopfield present his famous paper about continuous neural nets, showing how they mimic crystallization. His insight inspired me to study such things at Caltech two years later, where he sat on my dissertation committee.
While I studied various types of neural nets, my PhD thesis in physics and theoretical neuroscience explored real neurons, not abstract, artificial ones. In practical terms, instead of producing code to get grant money as AI researchers do, we theoretical neuroscientists had to explain evidence to get grant money. To most neuroscientists, evidence is more important than mathematical sense.
So, I found few friends when that dissertation used math to prove, in effect, that neuroscience was wrong. More specifically, I used big data and to show statistically why “neural noise” must actually be fine-grained information in disguise. Fortunately, for me, that crazy idea did find the two right friends.
One was electronics guru Professor Carver Mead, co-inventor of the integrated circuit. He gave my dissertation its best sound-bite: “One man’s noise is another man’s information.” The other was neural-net graybeard Terry Sejnowski. He invited me to present my dissertation to him, Zach Mainen and Francis Crick. Two years later, Mainen and Sejnowski experimentally confirmed my main prediction in an article that got thousands of citations.
After my postdoc, I moved back home for an industrial job in Silicon Valley. I worked my way up at various startups from a programmer, through staff scientist, to software architect and, ultimately, Silicon Valley’s first “chief algorithm officer.” In every role, I was a sole contributor, crafting my own database queries, writing my own code, creating my own graphs. I had a root password and my job was to tell the CEO and attorneys what was real. (On the side, I sometimes wrote for The Register, as a colleague of Cade Metz.)
That practice gave me lots of experience ranking and testing data-processing principles, in addition to what I already knew about brains and neurons. Those threads merged in a research paper — “Elastic Nanocomputation in an Ideal Brain” — that shows brains must be 3D physics engines.
My wife, Criscillia (who understands the informational structures of narrative and media), and I took two years off to lock into the permanent scientific record our further discovery about the informational interactions brains need. We found that human brains work thousands-fold faster than neuroscience notices, as they must in order to build trust in the senses, and are far more sensitive. I believe our 59-page essay, “Sensory Metrics of Neuromechanical Trust,” is the most scientific explanation of human trust there is. (As an example calculation, we compared the bandwidth of spoken words, about 11 bits/second, to the bandwidth of vibratory social signals, which flow a hundred-thousand-fold faster, in the megabit range.)
Here are two sections explaining brain-like computation for technologists, based (of course) on first principles.
The Quantization Fallacy
The quantization fallacy maintains that the only information that matters is that which is measured. That is to say, quantized and preserved. People who are good at rules and categories — like mathematicians and programmers — are especially vulnerable to this idea, even though it flies in the face of math itself.
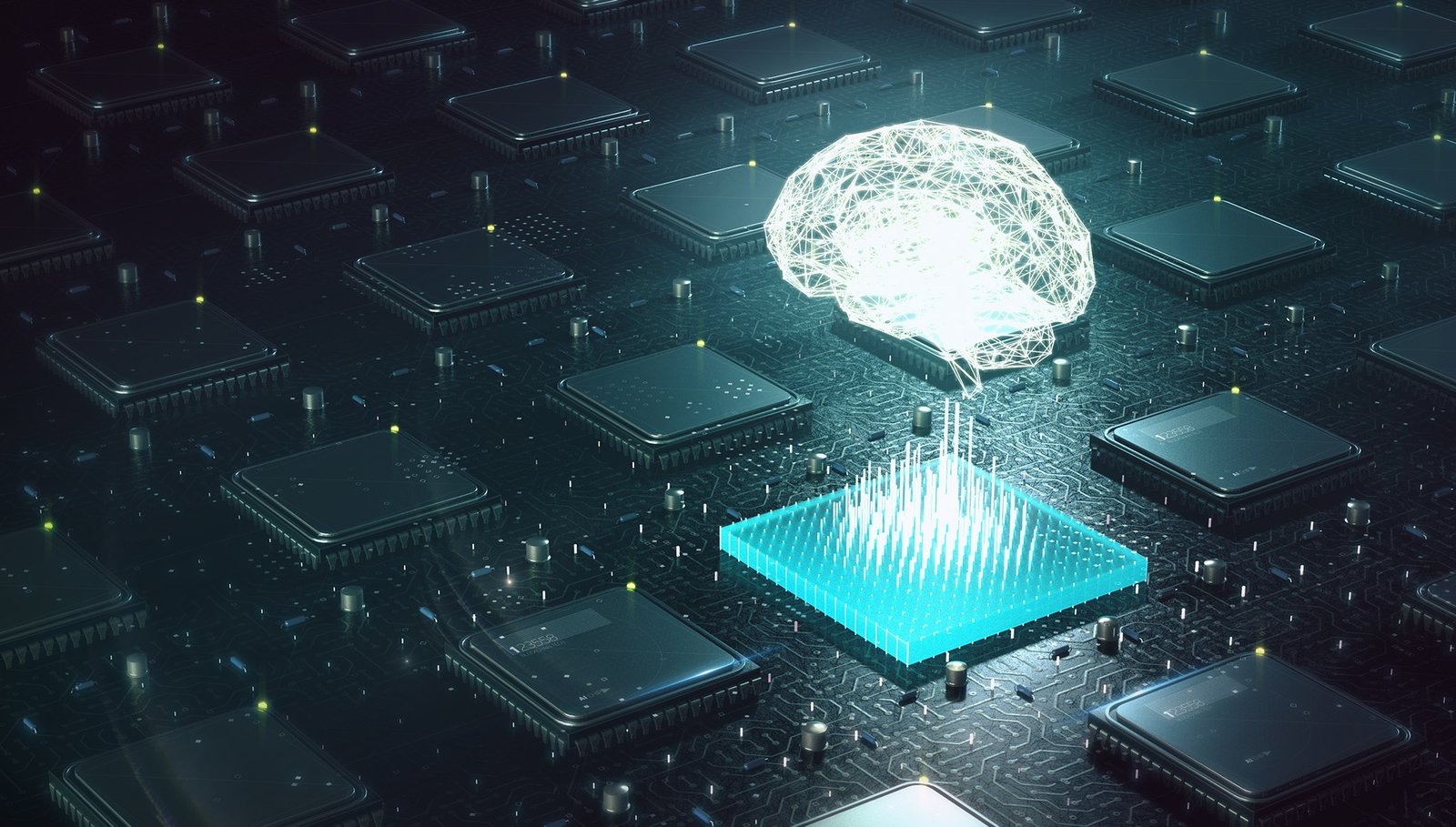
No concept in number theory is more basic than the distinction between real numbers and integers. The integers are countably infinite, the real numbers uncountably infinite — in fact, transcendental. Integers have “measure zero,” which means if the integers were somehow removed from the real number line, you couldn’t measure the difference. But no matter how many integers one has, one can’t reconstruct even a single real number from them.
If real values can’t be quantized and the real world is continuous, then those facts together impose an ironclad constraint on representation. They mean one cannot even, in principle, reconstruct a continuous reality from any fixed set of numbers, real numbers or not. So, the mere process of picking a specific spot in space or time, the very process of quantization itself, necessarily and irreversibly destroys information, the same way rounding-off does. Our brains may perceive a smooth, seamless world, but that’s because they hide the pixilation errors caused by neural pulses. The tradeoffs between real and integer, between analog and digital, are as subtle as quantum mechanics and similarly slippery.
When Claude Shannon wrote down the equations of information flow, he calculated real-valued information from real-valued probabilities. No one questioned or questions the fact that information can flow continuously on continuous waves (otherwise, we could neither see nor hear).
Shannon did his calculations using quantized messages. For deliberate, point-to-point communication, you want the same message you sent to appear at the far end of your information channel. To ensure that happens, you have to in a sense freeze-dry the message into some fixed form before transmission, whether in an envelope or a bit, so it doesn’t disperse and decay on the way.
The principle that information is natively continuous also applies to storing and calculating. Digital computers do use fixed bits, but vinyl disks and photographs (not to mention cave art) do not. Yet those store continuous slivers of real life, and analog circuits can process such slivers seamlessly. Yet those, like all quantized or recorded information, represent not just an infinitesimal portion of the real world, but the most malleable, systematically-biased and thus unreliable piece of it.
Recorded information isn’t really real, hence not completely trustworthy. That means “evidence,” measurements and tests are grossly overrated compared to basic mathematical principles.
Life and the Brain
In the beginning was life, that is self-regulation, plus self-replication. The process of self-regulation (homeostasis) ensures a creature ingests and expels just the right amount of what it needs, using built-in circuits that avoid both “too little” (in which case it seeks more) and “too much” (it backs off). If both extremes are possible in its world, then the creature will have both kinds of circuits. But if only one is likely — say, not enough sugar but never a surplus — then the creature doesn’t need hardware to avoid the surplus. So, instead of a two-sided regulation circuit, it uses a simple one-sided circuit seeking something rare — a circuit we might call “appetite,” which can easily fall into ruts if it learns the wrong things.
All of life runs on entropy, that is the diversity or scrambled-ness of combinations. Thermodynamics says entropy will always increase (become more scrambled) over time. But that only applies to a sealed-off system, away from any energy source. Lucky for us on Earth, we have the sun on one side and dark space on the other. That means life has energy to run those two basic operations, self-regulation and self-replication, both of which rearrange matter in ways that lower local entropy instead of raising it.
But there’s a catch. As Mickey Mouse learned in “The Sorcerer’s Apprentice,” once autonomous self-replication starts, it’s hard, if not impossible to stop. Furthermore, entropy-reduction mechanisms tend to accelerate toward singularities instead of dying out slowly. That simple observation says that life will cover the Earth eventually and then ever-fancier kinds of stuff will cover that. That is the same end-game of universal sameness envisioned by two geniuses in “Genius Makers.” Elon Musk envisioned an Earth covered by paper-clip factories, and Ilya Sutskever saw an Earth covered by Google offices. (In fact, most of Silicon Valley is already covered by a near-uniform coating of asphalt, concrete boxes and solar arrays.)
The next form of life was single-celled animals, that is creatures that move. Any animal’s most fundamental choice is to dial a balance between saving energy by staying put versus using energy to move elsewhere (say, to get resources or avoid damage). In thermodynamic terms, narrowing or focusing one’s search space lowers data entropy, while spreading, blurring or diffusing it raises data entropy.
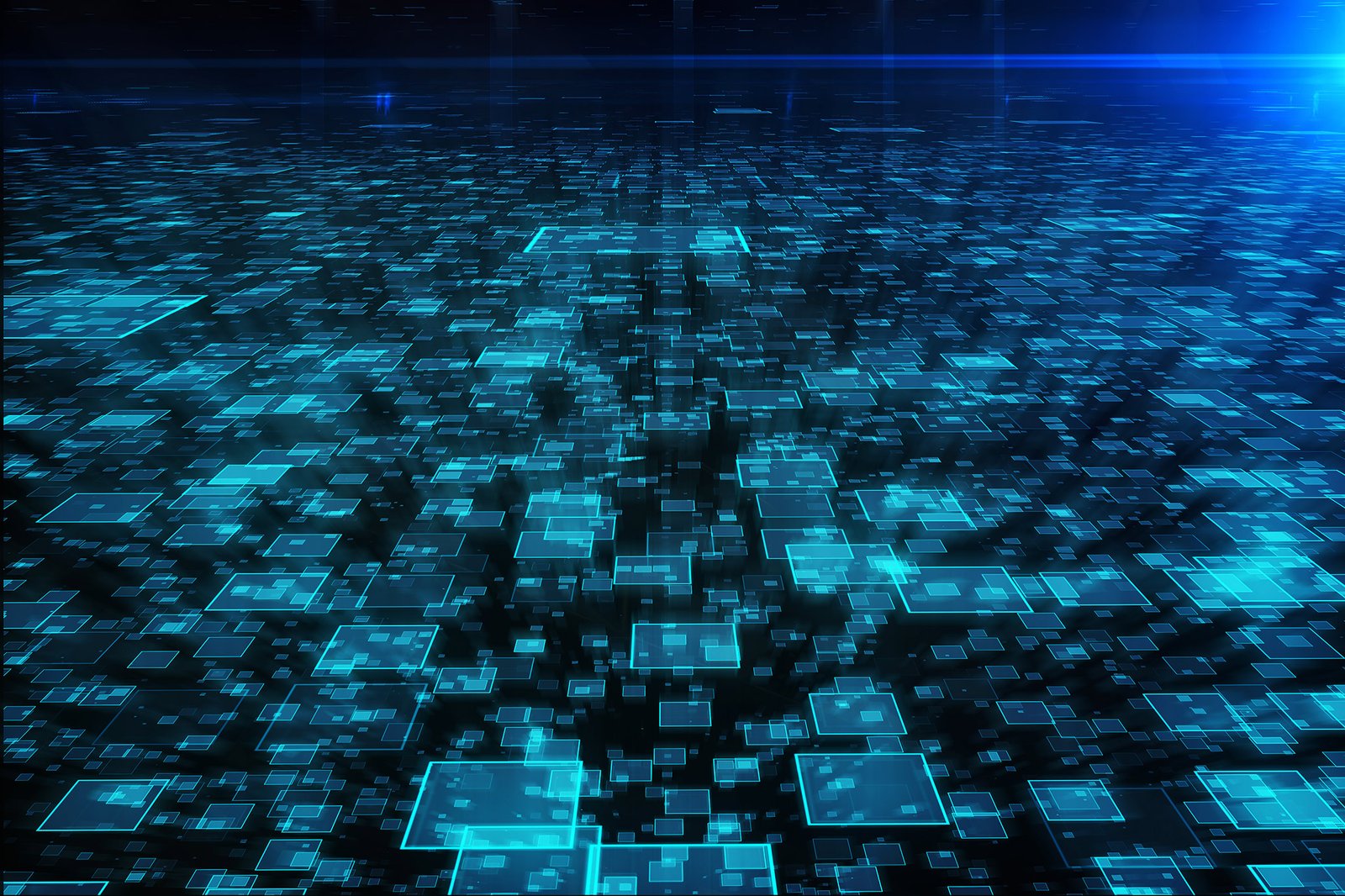
After animals came multi-celled animals and, eventually, vertebrates. Each more elaborate body structure came with more complex motor-control hardware, built out of and atop the older, simpler, more basic layers. Biological hardware evolves like the technological type, iteratively and incrementally, starting with metabolism, then vertebrate spines and then limbs made out of mini-spines attached to the main one, all of them meant to be wiggled with ever-increasing precision and elaboration. In such a real-time control system, memory and symbols have no place. Bandwidth is all.
Our immediate ancestors were quadrupeds, then primates, then bipeds. All of those bodies are made of bone and muscle, continuous and springy, not hard and hinged like robots. We bipeds, in particular, could run long distances to chase down prey because our loping gait can be so energy efficient. Mechanical and computational efficiency were our paleo superpowers, a far cry from the wastefulness of digital AI.
How could our ancestors be so efficient? Let’s pose the problem technologically. Suppose we had a biped “robot” with realistically elastic, anatomical tendons, muscles, joints and so on, like in the series “Westworld.” What kind of robot control would it need?
That robot brain would need to do two things: Make a picture of the world from its sensory input and then use that picture to control its body and world with output. That is, it needs a simulator to turn data into a world model, and a controller to turn the world model into motion.
First, the simulator, because if a creature can’t sense its shape or surroundings, there’s no point using muscles. As bodies contain solid, liquid, gas and in-between, the simulator needs to model all those states of matter — that is, to create both visual and felt 3D images of them in physically reasonable configurations and motions. In other words, a brain must contain a physics engine, a “visco-elastic simulator,” as part of its 3D-image-making (“tomography”) hardware, so it could synthesize either feelings of springy flow such as mucus or of hard brittle bone, each from a handful of neural pulses.
Such a gadget could simulate muscles and potentially learn to control them, probably as follows. Suppose the muscles are strung along a vertebrate spine like active rope, each tiny fiber tightening a bit from a motor-neuron pulse. The mechanical waves from those tightenings travel up and down the muscle bundle, occasionally bending and reflecting like sound does in cables. Sometimes, a passing pulse will knock loose a previously-tightened fiber or trigger a mechano-sensor to send the brain a pulse at just the right time. (Those pulses update the ongoing simulation.)
Such a simulator (e.g., brain) could synchronize those pulses to ring the muscles with pure tones or chords. The idea that a body’s squishy meat could sustain pure vibrations seems silly, but that’s because dead meat damps vibrations. But this would be active meat, whose activity exactly cancels the damping. Call it active anti-damping, in which new muscle firings restore vibrations’ lost energy in order to sustain a continuous, vibrating “carrier wave” that serves as an ongoing reference to the current body state. The metaphor is that of a supercollider, monitoring coherent vibrations and kicking them back into shape using specially-timed output pulses. This is the “innate machinery” Gary Marcus spoke of that brains use to make sense of space and time.
Here’s how hardware optimization works in brains. The higher the timing precision, the higher the physical precision. So, a brain operating with microsecond timing (e.g., temperature-stabilized) could potentially maintain a phase-coherence in spinal phonons (sound waves) beyond the ultrasonic into the megahertz range. All it takes is a circuit that learns to maximize the amplitude and frequency of the vibrations it reflects.
Such precision is thousands-fold higher than neuroscience ever looks for, so there is no experimental evidence of it yet. But such precision must be there, being dictated by both the laws of math and by the laws of information flow through space and time. There is simply no other way to move a piece of meat. It’s written in the physics. Neuromechanical vibrations are the only information channel with enough bandwidth to feel and control muscles efficiently. No fluid, chemical or electromagnetic channel comes close.
With such a simple structure, lots of functions come for free. Limb control results when high-frequency vibrations, dialed strong enough, aggregate into slower and larger ones. This down-conversion produces motions big enough to move a limb and slow enough to see. (Ordinary body tremor is halfway in between.) Thus, a simple, jelly-management simulator could control a vibrating body just by controlling the amplitude, frequency and specific phase of its physical “vibratory eigenmodes.”
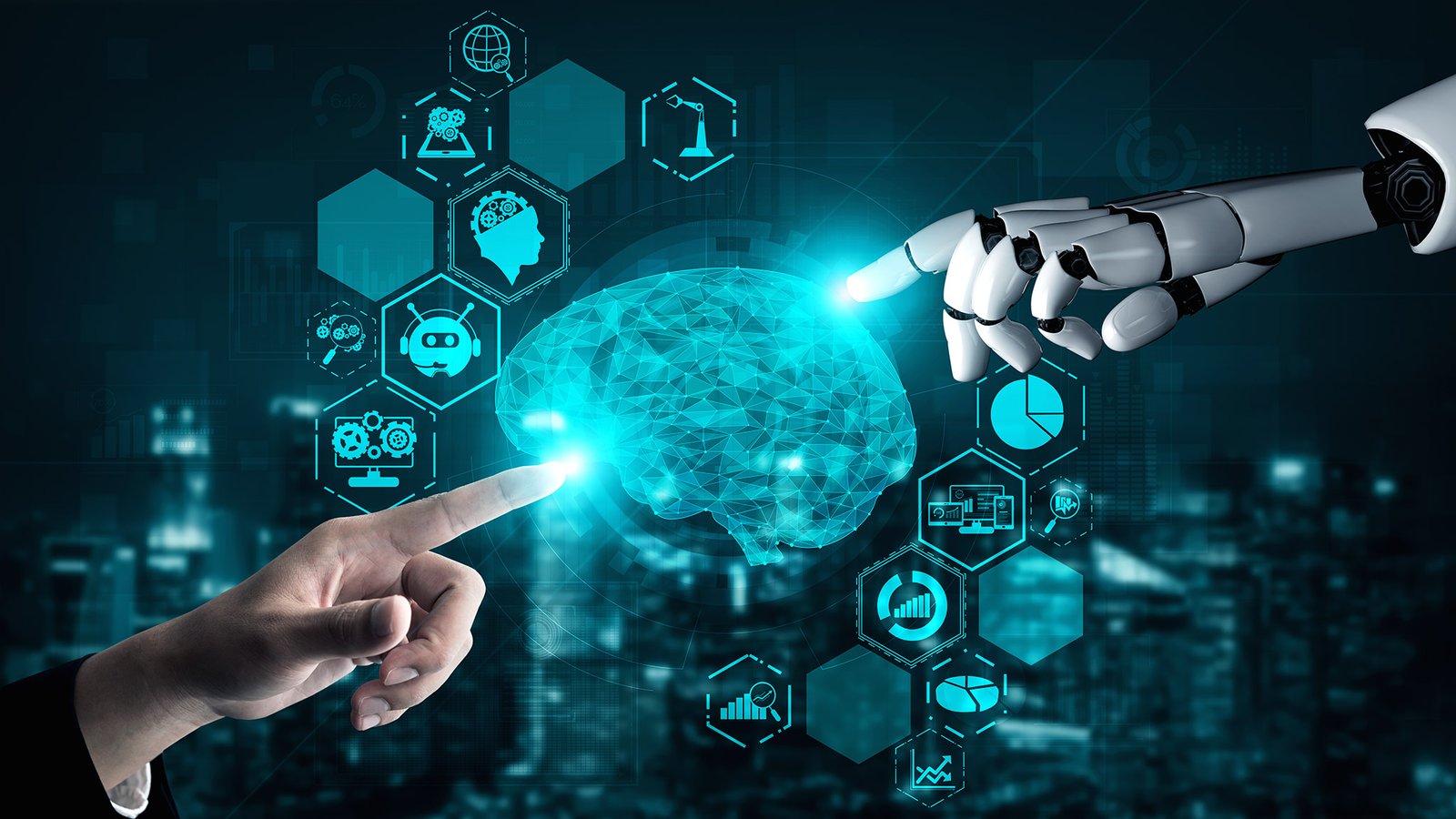
Spines vibrate at the highest frequencies when straight, so spinal straightening comes for free. Eyeballs are made of vibrating jelly, so vision comes for free. Deep sensations come from midline muscle groups, like those of sadness in the head, nausea in the gut and sex in the pelvis. Each locus has a different set of fluids, spastic contractions, sounds and sensations. With these native hardware modes, something like felt emotions come for free. As vibrating bodies naturally resonate in proximity, high-bandwidth social interaction comes for free. (Predicting, for example, that flocking birds synchronize their flight through their tiniest, fastest flutters first.)
When Analog Brains Meet Digital AI
All this means that human brains must be analog, not digital, fully continuous in 3D space and time. They can’t possibly be using finite-element simulation based on separate neurons, blocks or nodes. The mathematical requirements of tomography mean brains must calculate with tiny wavefronts moving through a kind of jelly, computing in the spaces inside and between neurons.
Once the vibrations are in place to move a 3D body through a 3D space, then quantized states like episodic memory, recognition and symbols can take hold. They would have to be made from continuum waves, like individual transistors can made from continuous silicon. But you can’t do the opposite, making a continuum from chunks.
Exposing sensitive brains to unnatural environments that hack their appetites and trust is hard on them. No creature evolved to resist what it wants, nor to constantly fend off deception — especially children, whose immature nervous systems are so sensitive to training data. Long-established principles of neuroscience hold that early learning and mis-learning matter. Exposing children to AI bots before they’ve learned real people can’t be good.
The Final Frontier
I know lots of people like the geniuses. I went to a university filled with crazy-smart people like them, so I know how much they trust that math is real. The good ones can’t stand paradoxes. Like many of the heroes in Cade Metz’s book, the ones with the most integrity eschew megabucks and impact in favor of living peaceful lives and building human-friendly tech. Nerds or not, they care more about humans than about shareholder value.
So, once those people realize brains are analog and hyper-sensitive, building their trust from subtle interaction, they’ll ditch the dumb idea that only metrics matter. They’ll ditch artificial intelligence whose main job is to fool us and exploit our trust. Then they’ll invent new tech that helps our brains instead.
Their voyage into data space and hyperspace will explore strange new worlds of analog vibrational control, like toroidal body maps. They’ll seek out new, life-enhancing tuning tools and new civilizing ways for humans to interact. They’ll boldly go where no technologist has gone before, into the uncharted blue ocean of analog human self-awareness and connection, as understood by laws of information physics. They’ll be the most important geniuses of all, and I can’t wait to collaborate with them.
*[The articles in this column present a set of permanent scientific truths that interlock like jigsaw pieces. They span physics, technology, economics, media, neuroscience, bodies, brains and minds, as quantified by the mathematics of information flow through space and time. Together, they promote the neurosafe agenda: That human interactions with technology do not harm either the nervous system’s function, nor its interests, as measured by neuromechanical trust.]
The views expressed in this article are the author’s own and do not necessarily reflect Fair Observer’s editorial policy.
Support Fair Observer
We rely on your support for our independence, diversity and quality.
For more than 10 years, Fair Observer has been free, fair and independent. No billionaire owns us, no advertisers control us. We are a reader-supported nonprofit. Unlike many other publications, we keep our content free for readers regardless of where they live or whether they can afford to pay. We have no paywalls and no ads.
In the post-truth era of fake news, echo chambers and filter bubbles, we publish a plurality of perspectives from around the world. Anyone can publish with us, but everyone goes through a rigorous editorial process. So, you get fact-checked, well-reasoned content instead of noise.
We publish 2,500+ voices from 90+ countries. We also conduct education and training programs
on subjects ranging from digital media and journalism to writing and critical thinking. This
doesn’t come cheap. Servers, editors, trainers and web developers cost
money.
Please consider supporting us on a regular basis as a recurring donor or a
sustaining member.
Will you support FO’s journalism?
We rely on your support for our independence, diversity and quality.